Business Intelligence in Healthcare Insights and Applications
Data is fundamentally reshaping the healthcare landscape, and business intelligence (BI), along with data analytics, are proving to be powerful engines for enhancing both operational efficiency and the quality of patient care. This article examines the fundamental components, key benefits, diverse use cases, and essential tools that define the application of business intelligence within the medical industry. Read on to gain insights into modern business intelligence concepts, particularly the empowering shift towards self-service analytics accessible directly by medical professionals at the point of care.
The blog discusses:
- definition of business intelligence in healthcare
- ways of information collection
- components of a BI system
- implementation challenges with solutions
- BI healthcare use cases
- future trends
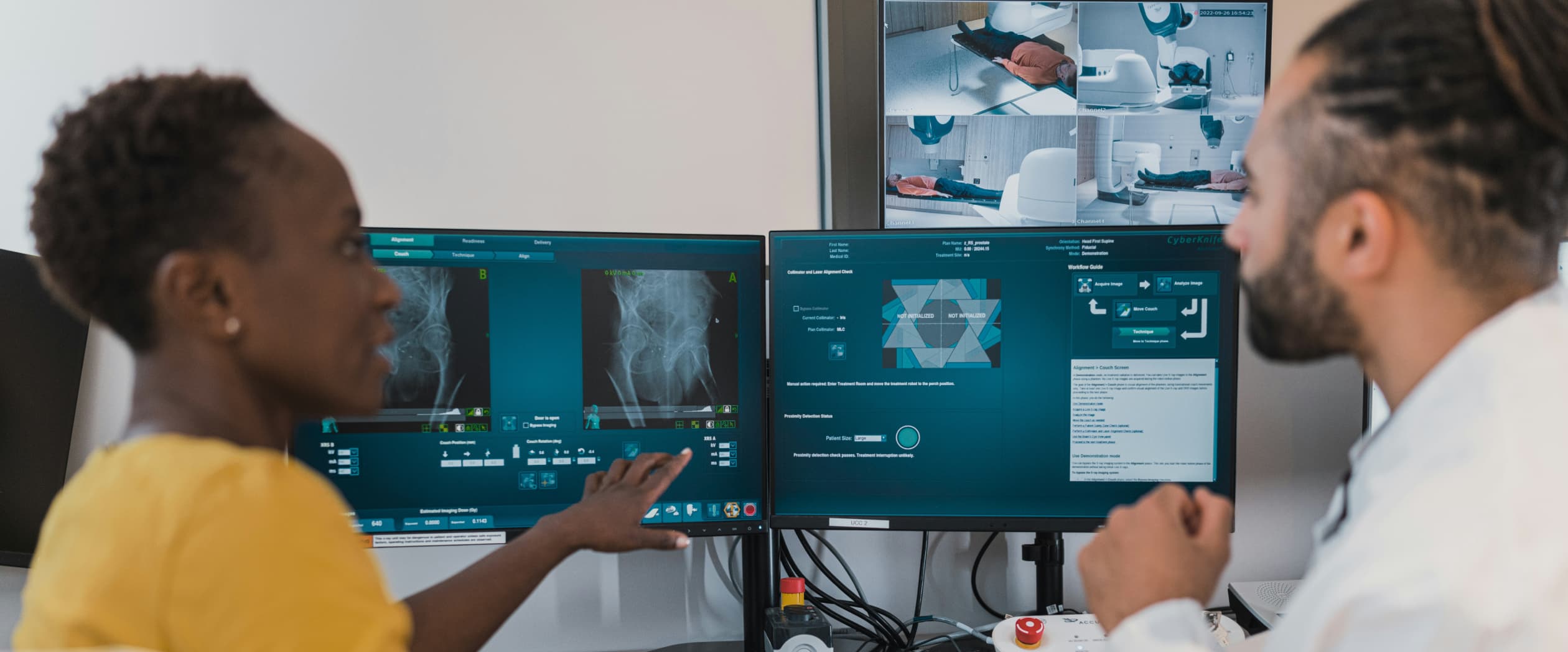
Table of Contents
The medical industry is undergoing a significant transformation powered by data, and business intelligence (BI) is the driving force of this evolution. In this article, we will look into the ways BI is revolutionizing healthcare operations and problem-solving, as well as explore the methodologies for data collection and the essential elements of contemporary business intelligence systems. We are also going to address the specific hurdles encountered when implementing business intelligence solutions leveraging Amazon Web Services (AWS).
Table of Contents
What is Business Intelligence in terms of Healthcare
At its core, Business Intelligence (BI) in healthcare represents the usage of data and software capabilities to access, analyze, and develop actionable insights, with the primary goal of improving business and clinical decisions. Its more recent versions, Next-Gen BI solutions, are focused on minimizing the time it takes for insight to be generated by leveraging real-time or near-real-time aggregation and analysis of structured and unstructured data sets. As a result, healthcare and life sciences organizations are able to improve patient care, enhance biopharma research and development, and streamline operations.
Why is BI Important in Healthcare
As previously mentioned, business intelligence, along with business analytics, leads the transformation of the way healthcare services operate, make decisions, and deliver care. There are a number of reasons this application plays a crucial role in the health services, namely:
- Improving Patient Care and Outcomes
Business intelligence tools like Amazon QuickSight allow medical professionals to analyze data from multiple platforms to proactively detect risks. For instance, these tools can help identify ICU patients at high risk of infection and initiate preventive care. Besides, business intelligence supports smarter test utilization, reduces readmission rates, and ensures timely and appropriate treatment based on the latest scientific findings. - Enhancing Research and Development
Modern BI solutions contribute to pharmaceutical R&D and health product innovation by allowing real-time analysis of structured and unstructured data. AWS and its partners support the development of Real-World Evidence (RWE) platforms, a feature that leads to accelerated research timelines, as well as helps uncover new treatment opportunities and enables predictive analytics. - Streamlining Operations and Improving Efficiency
Business intelligence aggregates financial, clinical, and transactional data, and therefore helps healthcare services remove outdated practices and optimize processes. The insights gathered from unified data help improve operational decisions and ROI, as well as increase organizational agility. - Personalized Medicine
BI provides platforms such as Deloitte ConvergeHEALTH Miner that help merge diverse datasets to determine optimal drug treatments based on individual characteristics, such as age, gender, disease progression, and lifestyle factors. This feature accelerates the development of personalized care. - Improving Clinician and Patient Experience
By giving real-time access to a summary of patient data, integrated business intelligence solutions improve physician processes. Amazon Q Business and other generative AI assistants free up physicians’ time so they may concentrate on patient care. Digital engagement techniques that provide a smooth experience throughout the care journey are powered by business intelligence on the patient side. - Ensuring Regulatory Compliance
BI solutions offered by APN Partners are in full compliance with strict regulatory requirements (e.g., HIPAA). The platforms it delivers, such as hc1, provide full security and compliance of sensitive health data, and support advanced analytics.
How Healthcare BI Collects Its Data
Data for business intelligence (BI) in healthcare is gathered from diverse sources in both structured and unstructured formats. This section will delve specifically into these varied origins of healthcare data and the methods employed for its collection
Electronic Health Records (EHRs)
The primary source of such information as patient data that contains demographics, medical history, diagnoses, medications, allergies, lab results, and clinical notes is the Electronic Health Records. These records are the groundwork for clinical problem-solving and patient care, and are managed digitally across hospital systems.
There are several ways through which the data is collected, specifically:
- Historical data exportation performed for retrospective studies or migration to new platforms;
- Real-time access through various APIs (e.g., SOAP, REST), and increasingly FHIR (Fast Healthcare Interoperability Resources) endpoints, which enable integration with analytics platforms;
- Applications implemented within EHR systems that ensure direct querying or interfacing with business intelligence tools.
Clinical Systems and Connected Medical Devices
Hospital systems usually operate in data-rich environments that involve numerous clinical systems and connected devices that continuously generate diagnostic and monitoring data. To illustrate, each patient bed typically connects 10-15 devices that track such indicators as vitals, respiratory function, and other real-time parameters.
There are four main contributors, which include:
- Laboratory Information Systems (LIS)
These systems manage test results like blood panels and cultures. - Picture Archiving and Communication Systems (PACS)
The contributors store diagnostic imaging such as X-rays, CT scans, and MRIs. - Telemetry devices and bedside monitors
Those are used for continuous real-time physiological monitoring. - Clinical video systems in specialized care settings
They are meant to capture video streams for later review or AI-based analysis.
Administrative, Financial, and Operational Systems
The main goal of these systems is to collect structured data that is used to track performance, revenue cycles, and resource utilization, while also supporting business intelligence initiatives that assess financial health and operational efficiency.
The systems use a few sources, such as:
- Billing platforms that store log information related to patient invoices, payments, and claims.
- Insurance claim systems are supposed to document reimbursements and denials.
- Scheduling tools are used for patient appointments and staff resource allocation.
- Supply chain systems perform for procurement, inventory management, and tracking logistics.
Patient Engagement and Communication Platforms
These types of platforms are used to collect data that reflects patients’ interaction with medical professionals, and be the source of insight into users’ behavior and preferences. The main goal of these systems is to help in developing personalized outreach and care plans.
The data sources for these platforms include:
- Patient portals
They capture secure messaging, appointment scheduling, and prescription refills. - Telehealth applications
These log virtual visit details and usage trends. - Mobile health apps
These applications record symptoms, medication adherence, and wearable device integrations.
Research and Real-World Data Sources
To develop relevant treatments and analyse real-world patient outcomes, healthcare services and life sciences firms leverage certain sources, some of which are:
- Clinical trials data
This type of information is collected from controlled studies that evaluate drug efficacy or treatment effectiveness. - Research registries
This is a source of disease-specific or treatment-specific patient information. - Real-World Evidence (RWE) platforms
They integrate patient outcomes outside of clinical trial settings.
Unstructured Data Sources
Unstructured data is the type of data that is rich in clinical meaning but difficult to comprehend without thorough processing, as it involves free-text and image-based content.
There are several key source examples, namely:
- Clinical notes
They are compiled by physicians and nurses as a form of documentation of patient observations and care plans. - Scanned documents
They involve intake forms, consents, and legacy paper records. - Emails and call transcripts
This data usually comes from support centers or communication logs that may contain sentiment or behavior signals.
Key Components of a Business Intelligence System in Healthcare
The effectiveness of Business Intelligence (BI) in healthcare hinges on a robust underlying system. In this section, we will review the essential components that enable the processing, storage, and analysis of collected data and help transform it into actionable insights for enhancing both patient care delivery and operational efficiency.
Data Aggregation
The foundational layer for healthcare business intelligence is the process of combining data from multiple sources into a unified format. This aggregation helps ensure consistency, reduces duplication, and enables holistic analysis across departments and data types. Just a couple of the services that support this holistic view of data are: AWS Lake Formation (an application that offers centralized access and policy-based data sharing), and platforms like Deloitte ConvergeHEALTH Miner (which facilitate the harmonization of clinical, operational, and research data into a common schema).
Data Integration
The next step after data aggregation is the process of transforming it into standardized formats, as it helps manage the diversity of data sources and reach compliance with healthcare standards such as HL7 and FHIR. There are services to help you achieve this: AWS Glue delivers serverless ETL pipelines, Amazon HealthLake ingests and converts health records into FHIR format, and platforms like hc1 enable integration of various kinds of data (e.g., clinical, lab, and financial data) at the patient level.
Secure Data Warehousing
The key to compliance with standards like HIPAA and other requirements, health information demands a high level of security. To achieve that, consider implementing solutions like Amazon Redshift, as they offer scalable and efficient querying of large datasets. Meanwhile, platforms like AWS Key Management Service (KMS) ensure encryption at rest with fine-grained access control to protect PHI.
Healthcare Information Management
Reliable business intelligence calls for robust data governance and quality, which is represented by clearly defined access permissions, supervision of data lineage, and metadata management. For that purpose, AWS Lake Formation helps enforce access controls and policies, while AWS Glue Data Catalog enables searchable indexing and minimizes data redundancy.
Real-time Analytics
In clinical environments, prompt insights can play a crucial role. In order to obtain this critical information, streaming tools such as Amazon Kinesis and Amazon MSK (Managed Streaming for Apache Kafka) capture live telemetry from devices and systems, and utilize dashboards that support such features as real-time problem-solving, alerts, and proactive care interventions.
Dashboards and Visualizations
Data interpretation and insights transmission hinge on visual tools that are provided by Amazon QuickSight, an application that allows medical professionals to compile dashboards connected to various health information sources. These dashboards help drill-down capabilities, deliver interactive filtering and storytelling features to support data-driven decisions.
Self-Service Data Exploration
Another requirement for business intelligence platforms is the ability to allow non-technical users to explore health information without additional guidance. Tools like QuickSight Q enable users to ask questions in natural language and receive real-time data visualizations. Additionally, scenario-based tools allow users to explore different outcomes and emphasize future trends through intelligent suggestions.
Integration with Workflows
The most proficient way to leverage business intelligence tools is to integrate them directly into medical professionals’ daily systems. For instance, EHRs, CRMs, or internal portals can be embedded with QuickSight dashboards to ensure that insights are promptly delivered at the point of care or decision, which helps reduce context switching and enhance workflow efficiency.
AI and ML for Enhanced Insights
Business intelligence capabilities can be transformed from characterization into forecasting and recommendations with the help of artificial intelligence. In this case, Amazon SageMaker serves as a means to build and deploy machine learning models for applications like risk prediction or resource optimization. This platform also enables applications such as risk prediction. Another app, Amazon Comprehend Medical, analyzes unstructured clinical text and distills it into meaningful entities, while Amazon Q in QuickSight uses generative artificial intelligence to automate insights, summarize patterns, and suggest relevant questions.
How AWS Helps Mitigate Common Business Intelligence Implementation Challenges
The implementation of Business Intelligence (BI) solutions in healthcare often presents significant challenges. However, by leveraging the robust capabilities of AWS and partnering with experienced vendors offering comprehensive healthcare solution development services, many of these challenges can be effectively mitigated or prevented.
BI implementation challenge | AWS-Based Solutions |
Data silos and lack of interoperability |
|
Data security and regulatory compliance |
|
Volume, velocity, and variety of data |
|
Legacy systems and technology consolidation |
|
Lack of data skills and business user adoption |
|
Integration with clinical workflows |
|
Demonstrating ROI and managing costs |
|
Data quality and trust |
|
Complexity of healthcare data models and terminology |
|
Future Trends in Healthcare BI
The landscape of Business Intelligence (BI) and business analytics in healthcare is in constant change, prompted by increasing demand for data-driven insights, including machine learning. In this section, we will explore some of the key future trends shaping how healthcare services will leverage business intelligence to enhance patient care, optimize operations, and drive innovation in the years to come.
Generative Business Intelligence and AI-Powered Insights
The development of tools is one of the main changes in data interpretation and communication. A prime example is the wide usage of artificial intelligence algorithms to autonomously analyze health information, detect patterns, and generate narratives or visualizations that explain the findings. This shift significantly minimizes manual effort and enhances insight clarity across clinical and operational teams, both of which heavily rely on business analytics.
Meanwhile, Generative Business Intelligence substantially streamlines the interactions between users and health information, as it uses natural language, automatically creates visualizations, and performs calculations, which ultimately simplifies the analysis. When it comes to specific tools, Amazon Q in QuickSight performs a variety of functions: it can suggest analytical paths, summarize results, build structured data stories, and present complex information understandable for the overall user experience.
Unifying Structured and Unstructured Data for Deeper Insights
Another recent trend is the integration of structured and unstructured data to achieve deeper insights. Both types of health information are critical: structured data (e.g., lab results, Electronic Health Records fields) is essential for analysis, and unstructured sources, such as clinical notes, medical images, research papers, and emails, provide valuable context that has often gone underutilized.
With the help of advanced AI, it is now possible to extract and interpret information from diverse data sources and achieve more comprehensive and context-aware analysis. In fact, the integration of various sorts of health information enables better diagnostics, treatment planning, and operational decisions.
Embedded and Analytics in Clinical Workflows
Clinical workflows are growing more efficient with the help of embedded analytics, an approach that delivers timely insights within the systems that are already used by medical professionals (e.g., EHRs and other clinical applications). The shift is significant: relevant data appears directly within existing interfaces without interrupting workflows, instead of requiring separate tools or reports.
The primary advantage of this method is that it provides access to patient trends, risk indicators, and operational metrics, which ultimately results in faster, context-specific problem-solving. To be more precise, if dashboards are embedded straight into EHRs, clinicians are provided with immediate visibility into patient and population health data with maximum accuracy without interrupting the workflow.
Self-Service Business Intelligence and Data Democratization
Data exploration is significantly more accessible to a wider range of users within healthcare services as a result of Self-service Business Intelligence. Now, clinicians and administrators can now generate reports, visualize trends, and ask data questions directly without external help in technical expertise.
This evolution fulfills multiple purposes, as it both accelerates problem-solving and reduces bottlenecks for data teams. For example, tools such as Amazon QuickSight Q allow for intuitive, self-guided analysis that doesn’t disrupt daily healthcare operations.
Cloud-First Business Intelligence Architecture and Interoperability
Healthcare analytics are moving towards more scalable, flexible, and efficient environments with the help of Cloud-first Business Intelligence architecture. Cloud platforms offer a vast variety of services that also include AI and machine learning, while reducing infrastructure overhead and improving agility.
Meanwhile, interoperability allows insights to be obtained from seeing all data together in one complete view. It also supports standards like FHIR, which simplifies this integration and ensures consistent sharing of health information across platforms. In terms of requirement compliance, cloud-based solutions offer HIPAA-compliant environments designed specifically for healthcare.
Personalized and Proactive Insights for Patient Care
Patient care is moving away from universal strategies towards more individualized approaches with personalized insights leveraging data analytics. In the present day, medical professionals can predict risks and guide timely interventions through real-time inputs (e.g., medical device readings, wearable data, clinical trials, genetic profiles, and lifestyle information).
What this achieves is a deeper understanding of individual patient needs and the development of tailored care plans. Business intelligence tools can proactively detect health issues and help clinicians intervene earlier, improve outcomes, and allocate resources more effectively.
Security, Governance, and Regulatory Compliance
Regulatory compliance, security, and governance become even more important as the use of business intelligence tools grows. This specific trend serves the purpose of protecting sensitive patient data while adhering to standards like HIPAA and GxP, which is relevant for cloud-based environments.
It involves a few key priorities: establishment of clear governance frameworks, definition of data ownership, and enforcement of usage policies. Business intelligence systems incorporate a variety of security tools, such as granular access controls, encryption, and audit trails to monitor and safeguard data activity. In the future, business intelligence platforms are expected to implement compliance into their architecture, a feature that will help organizations remain trustworthy, ensure accountability, and operate under regulatory standards.
Real-Time Insights for Operational Excellence
When it comes to operational performance in healthcare, real-time insights are indispensable for maintaining productive workflows. By leveraging real-time insights, organizations can monitor key metrics (e.g., patient flow, staffing levels, supply chain activity, and financial performance) directly.
Another benefit of this approach is that it helps organizations promptly detect inefficiencies, which, as a result, allows teams to adjust resources, resolve issues, and maintain smooth operations. The purpose of real-time insights is to enhance processes and minimize expenses while maintaining the standards of care quality.
Frequently Asked Questions
While business intelligence (BI) and data science are closely related, they are not identical in their scope. The former analyzes historical/current data to help organizations understand the processes and make better decisions via dashboards and reports. Data science, on the other hand, surpasses this by using advanced techniques like machine learning to find patterns, make predictions, and answer complex questions about the future, a hallmark of modern data science practices.
Healthcare business intelligence is built upon five key tenets, presented as follows:
- Data Integration
The unification of data from diverse sources like EHRs, clinical systems, billing, and patient engagement tools into a consistent, holistic view.
- Data Quality and Governance
Guarantee of data accuracy, consistency, and compliance with regulations (e.g., HIPAA) via robust data validation, access control, and stewardship practices.
- Timely and Actionable Insights
Prompt delivery of relevant analytics to support clinical decisions, operational adjustments, and strategic planning.
- User-Centric Accessibility
Provision of intuitive and accessible healthcare business intelligence tools for diverse roles (clinicians, administrators, executives) that enable user self-service exploration and decision support.
- Scalability and Flexibility
Adaptable healthcare business intelligence architectures (e.g., AWS-based solutions) and scalable infrastructure as a means of supporting growing data volumes and evolving healthcare needs.
Clinical analytics or business intelligence fulfill a number of functions, such as identification of high-risk patients, optimization of treatment plans, minimization of re-admission, and provision of personalized care. The main purpose of these tools is to improve operational efficiency from multiple perspectives, as they also streamline workflows, enhance resource allocation, monitor performance, and ensure compliance with regulations. As a result, these methods provide the groundwork for data-driven decisions that aim to address both organizational performance and patient care.
In Quizlet-style terms, the medical industry would use business intelligence to collect, analyze, and visualize data in order to enhance problem-solving, reduce costs, and enhance care delivery. Business intelligence provides data unification and prompt analysis that helps improve data-driven decisions in various environments (e.g., clinical, operational, and financial areas). The industry will also utilize business intelligence to maintain personalized treatments, identify patient risks more efficiently, optimize resource allocation, and accelerate research and development by receiving relevant insights. Finally, business intelligence helps organizations reveal inefficiencies and support compliance with data security and privacy regulations, which significantly streamlines workflows.